The Goal – Advance Personalized Medicine
BioVariance GmbH focuses together with a global player from the pharmaceutical industry on advancing research in personalized medicine. Due to the outstanding cooperation and the excellent expertise of both partners, a new categorization of tumor indications will be available for the pharma and healthcare sector. The new categorization based on the individual characteristics of the tumor tissue shall provide a deeper understanding of disease mechanisms and further allow a more individual diagnosis and a more targeted and personalized therapy for affected patients prospectively.
Challenges
The rapid development of technologies for the biomedical and pharmaceutical research enable the generation of complex interdisciplinary data sets. Within the scope of this project, next-generation sequencing (NGS) and innovative optical measurement techniques were applied to characterize tissue sections to newly categorize the tumor indication of interest. Due to our broad expertise in Big Data related challenges, such as data processing, exploratory statistics and machine learning, the BioVariance GmbH was assigned to perform preprocessing of the datasets and to develop and evaluate the clustering models. To make both NGS and tissue characteristics data sets comparable and to correct for a possible bias caused by the new optical measurement technique, normalization of each data set was mandatory. The challenge was to find an appropriate normalization strategy that fulfilled both requirements. In addition, normalization should not produce spurious correlations within the data. Therefore, several normalization strategies were selected and tested. To ensure the absence of spurious correlation within the normalized data, the coefficient of variation [1] and partial correlations were determined. Finally, the best normalization strategy was chosen taking all three criteria (comparability of data, correction of bias, absence of spurious correlation) into account. Thus, the data scientists ensured, that the linear correlations between two variables of the data set (visualized by the scatterplot matrix) were not due to spurious correlations. The scatterplot matrix, however, did not show any clusters in the pairwise comparison of variables (in contrast to the scatterplot matrix from test data presented in Fig. 1). Consequently, the next step comprised a principal component analysis (PCA). For this purpose, the normalized tissue characteristics required a Box-Cox transformation, since none of these variables followed a normal distribution.
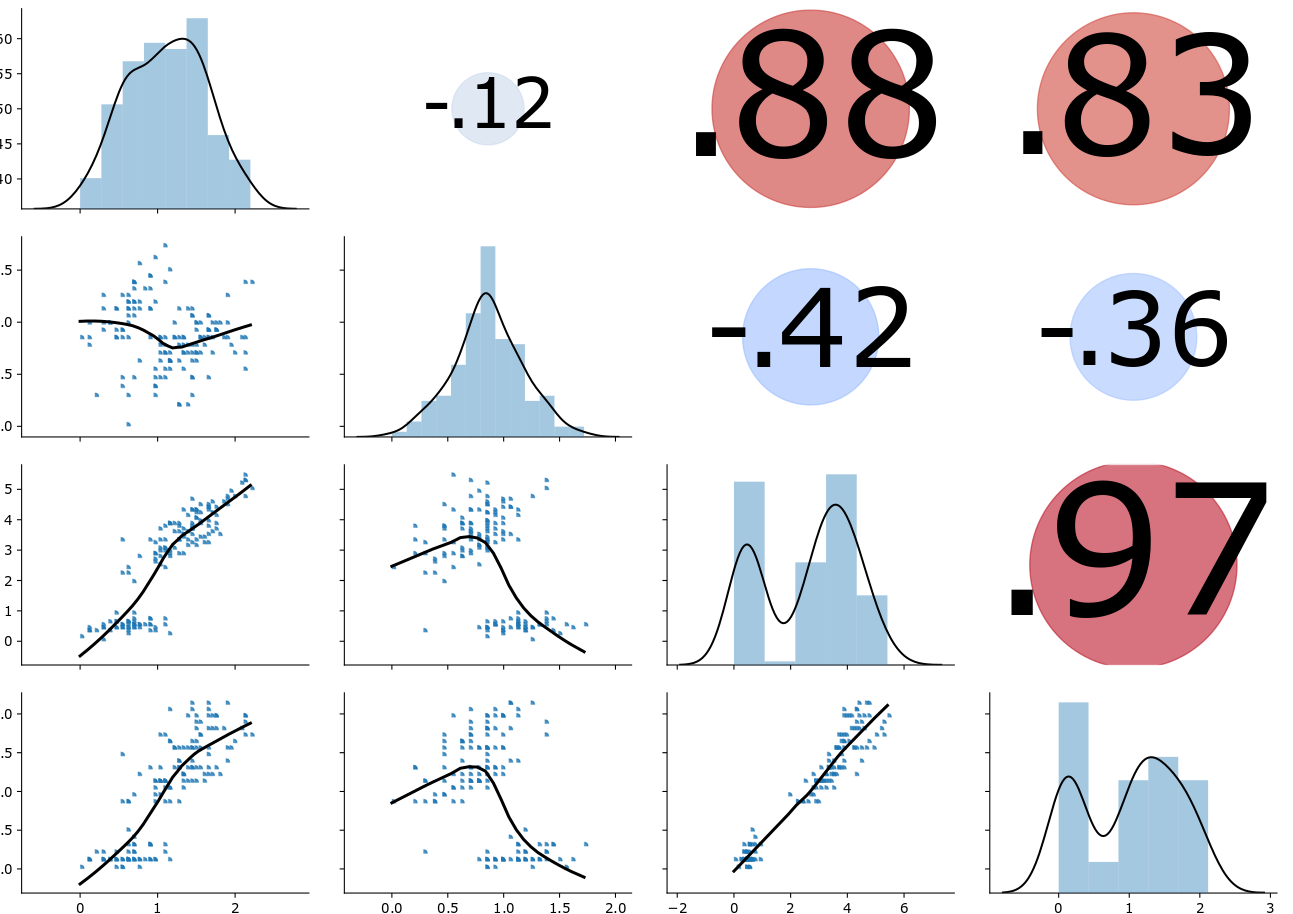
The road to success
Within the scope of this project, the team of data analysts at the BioVariance GmbH relied on descriptive statistics including state-of-the-art algorithms and exploratory data analysis to uncover differences, correlations and clusters in the data.
No clusters were observable in the scatterplot matrices. Consequently, a PCA was enriched by the Marchenko-Pastur distribution to find the discriminating factors. PCA reduces the number of variables in a data set by searching linear combinations, that describe the highest degree of variance – the so-called principal components (PCs). These PCs are the new variables that have the potential to visualize inherent clusters. The first PC describes the largest ratio of variance in the data, the second PC the second largest, etc. They construct an orthogonal coordinate system, of which the axes point into the direction of highest variance. Plotting the first two or three PCs is often sufficient to visualize clusters.[2] By means of the Marchenko-Pastur distribution the number of PCs carrying discriminating information was determined.[3] In this case, the first PC was identified as the only significant factor.
To confirm the visual result from the PCA, several clustering algorithms (hierarchical clustering using Manhattan distance, k-Means and PAM algorithms as well as dbscan) were
performed. Applying two parameters (e.g. the so-called silhouette-width) that describe the quality of cluster assignment for each patient, the most differentiated clusters were identified.
These newly discovered groups could be visualized and reinsured by labeling them in the PCA-Biplot (see Fig.2).
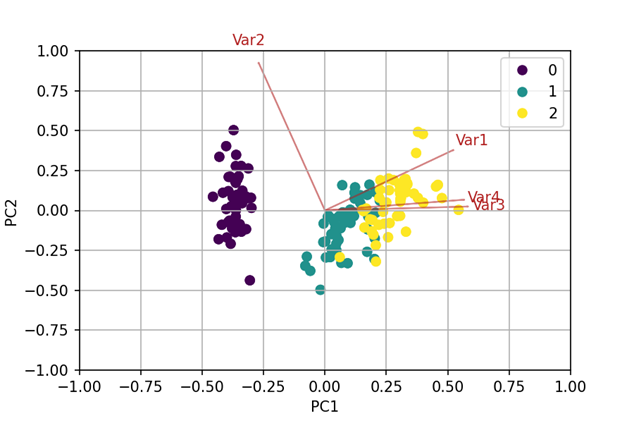
An established categorization which is based only on gene expression was compared to the new categorization. The results of this comparison were visualized by a heatmap representation. The two categorizations could be clearly distinguished from each other. In this study, the gene expression data of the tumors were applied additionally to identify biochemical processes responsible for the tissue characteristics.
The results from this successful cooperation were published in a high impact cancer research journal. They will strongly support the development of personalized therapy strategies.
If you are interested in a targeted extraction of information from your data set, too, then do not hesitate to contact us and request support of our experts.
Contact Person
Helen Schneider | Sales and Distribution
helen.schneider@biovariance.com